tf是坐标变换、位姿,用于表示两个坐标系间变换值,它关联的两个坐标系有严格的前后关系。“坐标系2-->坐标系1的tf”描述了一个从坐标系2到坐标系1的tf。举个例子,“odom-->map的tf”,如果是a2是odom坐标系下坐标,a1是map坐标系下坐标,那a1 = tf * a2。
一、tf树
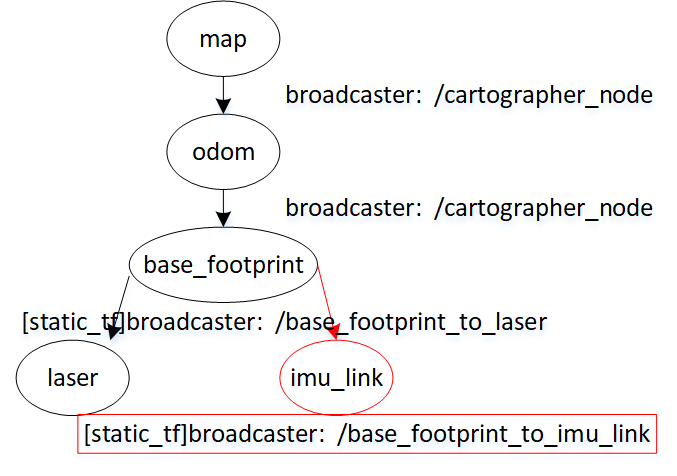
- map。地图坐标系。根坐标系(Fixed Frame),与机器人所在的世界坐标系一致。
- odom。里程计误差坐标系。cartographer发布。根据里程计读数算出的机器人理论位置(SLAM运动方程)和实际位置之间误差,这些误差形成的坐标系。机器人运动开始,odom和map坐标系是重合的,随着时间推移可能不重合了,而出现的偏差就是里程计的累积误差。所以,如果odom器件非常精准,精准到不会有噪声,那么odom-->map的tf就是0,map和odom这两坐标系一直重合。这有个疑问,cartographer不用里程计器件时,怎么得到odom-->map的tf?——它就是最新子图经过Global SLAM得到的全局位姿和该子图AddRangeData模块算出的初始位姿的差值,见函数ComputeLocalToGlobalTransform。
- base_footprint。机器人底盘坐标系,与机器人中心重合
- laser。激光雷达坐标系。与激光雷达的安装点有关,其与base_footprint的tf为固定的。相对map坐标系,它就是激光雷达在map的实时坐标。
- imu_link。IMU坐标系。与IMU器件的安装点有关,其与base_footprint的tf为固定的。相对map坐标系,它就是IMU器件在map的实时坐标。由于使用cartographer时,默认不使用IMU,所以用了红框。
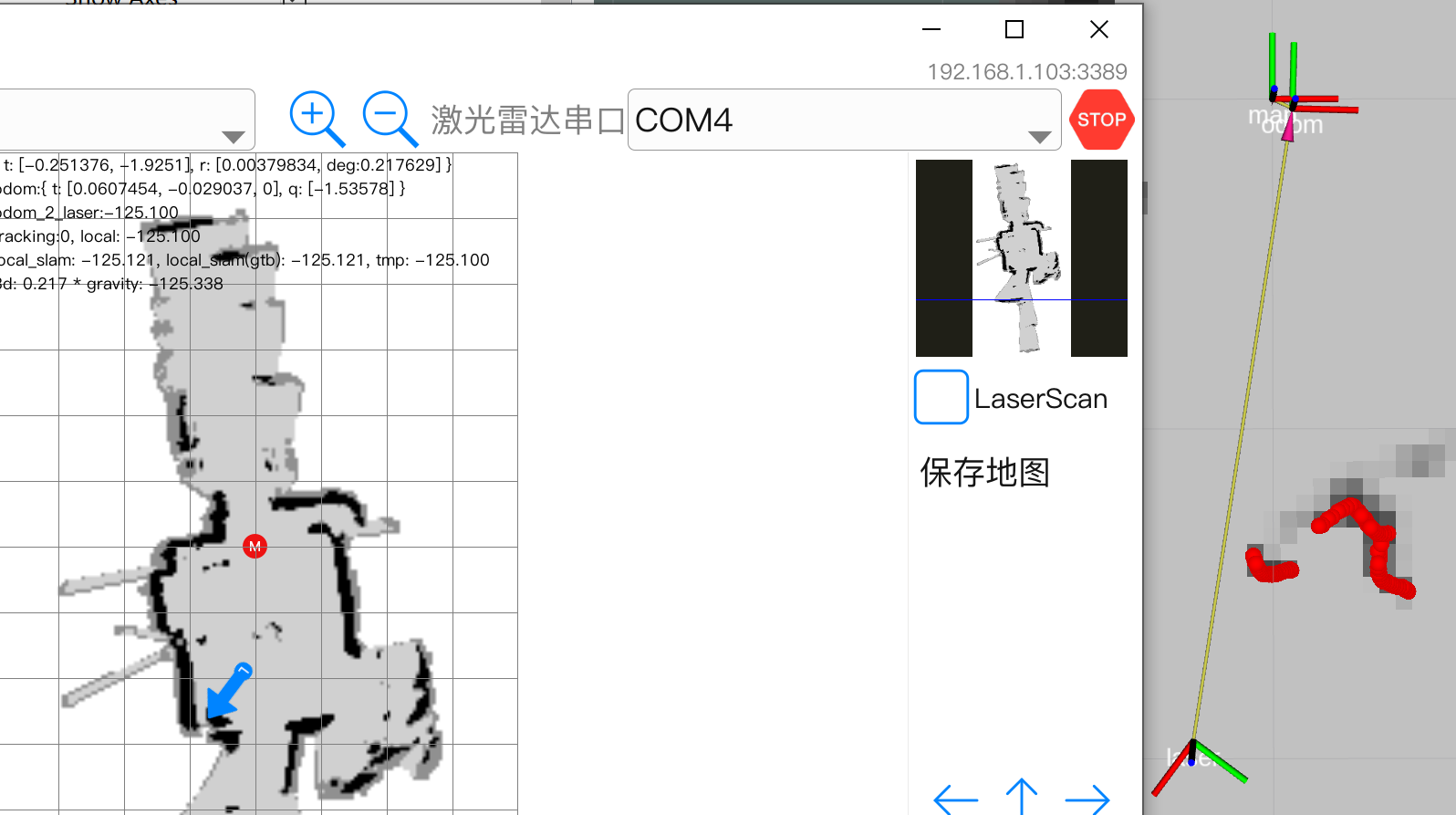
在图2右侧,rivz显示了map、odom、laser坐标系位置关系。机器人离起始点已差不多2米,Global SLAM算出里程计累积误差,即odom和map之间tf是{t:[0.0607454m, -0.029037m, 0}, deg[-1.53578度}。
二、Node::PublishLocalTrajectoryData
图1所示,cartographer_node节点发布odom-->map、base_footprint-->odom坐标变换,具体是在PublishLocalTrajectoryData。除这两个坐标变换,还发布“scan_matched_points2”话题,该活题的消息类型sensor_msgs::PointCloud2。在深入PublishLocalTrajectoryData前,让先看下正使用的lua配置,以及一次轨迹数据LocalTrajectoryData。
2.1 使用的lua配置、LocalTrajectoryData
<cartographer>/revo_lds.lua options = { map_frame = "map", tracking_frame = "base_footprint", published_frame = "base_footprint", odom_frame = "odom", provide_odom_frame = true, pose_publish_period_sec=5e-3, }
LocalTrajectoryData结构,它由两部分组成,节点(Node)固定就不变的local_slam_data,其它则会按当时状态实时计算。可近似认为一个节点(Node)对应一帧点云,一次LocalTrajectoryBuilder2D::AddRangeData后会生成一个节点,也就是说,一次AddRangeData后,PublishLocalTrajectoryData得到的LocalTrajectoryData中的local_slam_data才会发生改变。
class MapBuilderBridge { struct LocalTrajectoryData { // Contains the trajectory data received from local SLAM, after // it had processed accumulated 'range_data_in_local' and estimated // current 'local_pose' at 'time'. // local_pose指局部位姿,LocalSlamData存储着和局部位姿相关的三个变量。 struct LocalSlamData { // 计算local_pose的零时刻。 ::cartographer::common::Time time; // 零时刻时的local_pose。 ::cartographer::transform::Rigid3d local_pose; // 零时刻时在odom坐标系下的点云。 ::cartographer::sensor::RangeData range_data_in_local; }; std::shared_ptr<const LocalSlamData> local_slam_data; cartographer::transform::Rigid3d local_to_map; std::unique_ptr<cartographer::transform::Rigid3d> published_to_tracking; TrajectoryOptions trajectory_options; }; };
2.2 PublishLocalTrajectoryData
cartographer_node启动时会创建个间隔pose_publish_period_sec(5毫秒)的定时器,定时器函数PublishLocalTrajectoryData。
void Node::PublishLocalTrajectoryData(const ::ros::TimerEvent& timer_event) { absl::MutexLock lock(&mutex_); for (const auto& entry : map_builder_bridge_.GetLocalTrajectoryData()) { const auto& trajectory_data = entry.second;
for中"auto"表示的类型是std::unordered_map<int, MapBuilderBridge::LocalTrajectoryData>,trajectory_data类型是MapBuilderBridge::LocalTrajectoryData,一个LocalTrajectoryData表示GLOBAL SLAM后的一个节点的某次数据。
LocalTrajectoryData有4个字段,local_slam_data和节点的局部位姿相关,在MapBuilderBridge::OnLocalSlamResult生成,生成时机是在Global SLAM新生成此节点时。由于定时器间隔很短,会发生新节点还没到,定时器又被执行,执行这些时local_slam_data数值是不会变的。其它3个字段,则是此刻的GetLocalTrajectoryData()按当前状态生成。注意local_to_map,它就是“一、tf树”中的“odom-->map的tf”。
auto& extrapolator = extrapolators_.at(entry.first); // We only publish a point cloud if it has changed. It is not needed at high // frequency, and republishing it would be computationally wasteful. if (trajectory_data.local_slam_data->time != extrapolator.GetLastPoseTime()) { if (scan_matched_point_cloud_publisher_.getNumSubscribers() > 0) { // TODO(gaschler): Consider using other message without time // information. carto::sensor::TimedPointCloud point_cloud; point_cloud.reserve(trajectory_data.local_slam_data->range_data_in_local .returns.size()); for (const cartographer::sensor::RangefinderPoint point : trajectory_data.local_slam_data->range_data_in_local.returns) { point_cloud.push_back(cartographer::sensor::ToTimedRangefinderPoint( point, 0.f /* time */)); } scan_matched_point_cloud_publisher_.publish(ToPointCloud2Message( carto::common::ToUniversal(trajectory_data.local_slam_data->time), node_options_.map_frame, carto::sensor::TransformTimedPointCloud( point_cloud, trajectory_data.local_to_map.cast<float>())));
发布话题消息,话题名:“scan_matched_points2”,消息类型:sensor_msgs::PointCloud2。node_options_.map_frame值是map,也就是说,消息中的frame_id填的是map,不是odom。点云数据来自local_slam_data中的range_data_in_local,但range_data_in_local存的是odom坐标系的坐标,为转换到map,须要乘上trajectory_data.local_to_map。
导航时,障碍层计算代价地图会订阅这话题。
} extrapolator.AddPose(trajectory_data.local_slam_data->time, trajectory_data.local_slam_data->local_pose); } geometry_msgs::TransformStamped stamped_transform; // If we do not publish a new point cloud, we still allow time of the // published poses to advance. If we already know a newer pose, we use its // time instead. Since tf knows how to interpolate, providing newer // information is better. const ::cartographer::common::Time now = std::max( FromRos(ros::Time::now()), extrapolator.GetLastExtrapolatedTime()); stamped_transform.header.stamp = node_options_.use_pose_extrapolator ? ToRos(now) : ToRos(trajectory_data.local_slam_data->time); // Suppress publishing if we already published a transform at this time. // Due to 2020-07 changes to geometry2, tf buffer will issue warnings for // repeated transforms with the same timestamp. if (last_published_tf_stamps_.count(entry.first) && last_published_tf_stamps_[entry.first] == stamped_transform.header.stamp) continue; last_published_tf_stamps_[entry.first] = stamped_transform.header.stamp; const Rigid3d tracking_to_local_3d = node_options_.use_pose_extrapolator ? extrapolator.ExtrapolatePose(now) : trajectory_data.local_slam_data->local_pose; const Rigid3d tracking_to_local = [&] { if (trajectory_data.trajectory_options.publish_frame_projected_to_2d) { return carto::transform::Embed3D( carto::transform::Project2D(tracking_to_local_3d)); } return tracking_to_local_3d; }(); const Rigid3d tracking_to_map = trajectory_data.local_to_map * tracking_to_local;
在这个等式中,“map”指的是/map,“local”指的是/odom,“tracking”指的是base_footprint。
if (trajectory_data.published_to_tracking != nullptr) { if (node_options_.publish_to_tf) { if (trajectory_data.trajectory_options.provide_odom_frame) {
在launcher,provide_odom_frame是true,将发布两种tf。
std::vector<geometry_msgs::TransformStamped> stamped_transforms; stamped_transform.header.frame_id = node_options_.map_frame; stamped_transform.child_frame_id = trajectory_data.trajectory_options.odom_frame; stamped_transform.transform = ToGeometryMsgTransform(trajectory_data.local_to_map); stamped_transforms.push_back(stamped_transform);
发布odom-->map的tf。变换值是trajectory_data.local_to_map。
stamped_transform.header.frame_id = trajectory_data.trajectory_options.odom_frame; stamped_transform.child_frame_id = trajectory_data.trajectory_options.published_frame; stamped_transform.transform = ToGeometryMsgTransform( tracking_to_local * (*trajectory_data.published_to_tracking)); stamped_transforms.push_back(stamped_transform);
发布base_footprint-->odom的tf。变换值是tracking_to_local * (*trajectory_data.published_to_tracking)。
tf_broadcaster_.sendTransform(stamped_transforms); } else { ... tf_broadcaster_.sendTransform(stamped_transform); provide_odom_frame==false,只发布一种坐标变换。map-->base_footprintf。很少会有应用让进这个入口。 } } if (node_options_.publish_tracked_pose) { ::geometry_msgs::PoseStamped pose_msg; pose_msg.header.frame_id = node_options_.map_frame; pose_msg.header.stamp = stamped_transform.header.stamp; pose_msg.pose = ToGeometryMsgPose(tracking_to_map); tracked_pose_publisher_.publish(pose_msg);
很少会有应用进这个入口。
}' } } }
在PublishLocalTrajectoryData,由于满足条件publish_to_tf==true,于是要发布坐标变换。至于发布什么坐标变换,取决于provide_odom_frame。
provide_odom_frame==true,会发布两种坐标变换。默认,也是launcher正在使用的。
- header.frame_id=map(配置变量map_frame值)、child_frame_id=odom(配置变量odom_frame值)
- header.frame_id=odom(配置变量odom_frame值)、child_frame_id=laser(配置变量published_frame值)
provide_odom_frame==false,只发布一种坐标变换。
- header.frame_id=map(配置变量map_frame值)、child_frame_id=laser(配置变量published_frame值)。
激光雷达设备会发布个“scan”话题,它的frame_id是“laser”,那它怎么融入tf坐标树?——雷达和机器人中的相对位置是固定的,即laser坐标系和base_footprint是固定的,于是上层会主动发一个laser-->base_frootprint的static-tf,到此laser也融入了tf树。
三、配置
cartographer用*.lua存储配置,分到两个目录。
- <apps-res>/data/core/cert/cartographer/configuration_files。存储cartographer部分的配置。
- <apps-res>/data/core/cert/cartographer_ros/configuration_files。存储cartographer_ros部分的配置。注意当中有个revo_lds.lua,它会重载掉写在其它文件中的配置,包括<apps-res>/data/core/cert/cartographer中的。
运行cartographer_node节点时,须要提供“-configuration_basename”参数,rose_ros指向了<apps-res>/data/core/cert/cartographer_ros/configuration_files/revo_lds.lua。该lua文件除了提供名为“options”的配置块,还可以重载掉写在其它文件中配置。
运行cartographer_occupancy_grid_node节点不须要额外指定一个主lua文件。 <apps-res>/data/core/cert/cartographer_ros/configuration_files/revo_lds.lua ------ ...... TRAJECTORY_BUILDER_2D.submaps.num_range_data = 35 TRAJECTORY_BUILDER_2D.min_range = 0.08 -- RPLIDAR A1M8 [0.15, 12] TRAJECTORY_BUILDER_2D.max_range = 8. TRAJECTORY_BUILDER_2D.missing_data_ray_length = 1. TRAJECTORY_BUILDER_2D.use_imu_data = false .......
按惯例,对2d slam,以上配置应该出现在cartographer目录下的trajectory_builder_2d.lua。
<apps-res>/data/core/cert/cartographer/configuration_files/trajectory_builder_2d.lua ------ TRAJECTORY_BUILDER_2D = { use_imu_data = true, min_range = 0., max_range = 30., min_z = -0.8, max_z = 2., ... submaps = { num_range_data = 90, ... }, }
在trajectory_builder_2d.lua,设置了use_imu_data=true,即slam时会使用IMU设备得到实时角度速。但是,有着更高优先级的revo_lds.lua设置了“TRAJECTORY_BUILDER_2D.use_imu_data = false”,最终运行时采用use_imu_data = false,即slam时没有IMU设备得到实时角度速,要由timed_pose_queue_计算瞬时角速度。
同理,控制每张子图最多画多少个点云的num_range_data也由90变成35。